Tackling Fairness in Research
Author: Marshall V. King
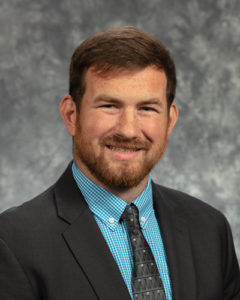
John P. Lalor – Assistant Professor in IT, Analytics and Operations
Breaking Bias: Tackling Fairness in Machine Learning Research
A research paper accepted for publication will showcase the work of Notre Dame professors and a doctoral student on fairness in machine learning.
John P. Lalor, Assistant Professor in IT, Analytics, and Operations at the Mendoza College of Business, and Ahmed Abbasi, the Joe and Jane Giovanini Professor of IT, Analytics, and Operations and Academic Director of the Ph.D. Program in Analytics, are co-authors with Kezia Oketch, a PhD student studying analytics and machine learning, and two others.
The paper titled “Should Fairness be a Metric or a Model? A Model-based Framework for Assessing Bias in Machine Learning Pipelines” will be published by ACM Transactions on Information Systems.
Perspectives on Fairness, Data Sparsity, and Predictive Model Performance
Lalor teaches students data analytics, particularly using the Python programming language as a tool. His research has focused on natural language processing algorithms. He has studied the development and competency of the models, but more recently has also assessed fairness and how to quantitatively measure it. Oketch and Abbasi supported that work, which offers a new perspective on research in the field of whether an algorithm is fair.

Ahmed Abbasi – Joe and Jane Giovanini Professor of IT, Analytics, and Operations
If machine learning utilizes an algorithm to determine whether someone is awarded or denied a bank loan, humans have traditionally been relied on to determine fairness. Assessing one dimension, such as age, gender, or ethnicity is relatively easy, but it’s far more difficult to weigh all those factors together.
“You’ll get more dramatic shifts in the results, just given the nature of the data that you have available, so you’ll get what looks like an extremely unfair model, but it’s more a function of the sparsity of data to get a proper distribution than unfairness,” said Lalor. “What we propose is a way to think about this fairness less as a measure of the outputs based on this demographic split and more as trying to predict the performance of the model as a function of the available demographic information that we have.”
The researchers can then see whether the algorithm is wrong, create a confidence score, and calculate an error.
“Now we can build a straightforward, easy-to-interpret regression model to predict the error as a function of those demographic attributes,” said Lalor.
Unveiling the Fairness Equation
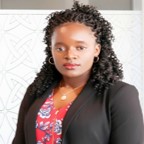
Kezia Oketch – PhD Student in Analytics
Those can all be inputs into a model to predict error. “Then we can get an interpretable output that assigns a weight to each of the demographics representing how wrong the model’s predictions are expected to be given particular setups of those demographics. So it takes care of the multi-dimensional issue because they all fit into a single model. Now we have one comprehensive look at performance concerning these demographics.”
The model can be used in various decision pipelines. It could assess how fair decisions are in rounds of a hiring process or healthcare. The researchers’ use of their model generated results that were more calibrated down the pipeline. “So if it’s, for example, biased against the elderly in the first stage, we would expect that same directionality of bias to be present in the second stage,” said Lalor. “That gives individuals more visibility into the process, and they can plan for certain outcomes if they’re implementing this in a production environment.”
A lot of research has been done in the data analytics field on assessing bias. “But to the best of our knowledge we are the first to take this modeling approach as opposed to a more metric-based approach,” said Lalor.
Oketch played a key role in the paper. “She did a nice job of running regressions, stress testing those regressions to make sure the results were robust across different configurations to confirm the results. And she was very helpful with writing text and producing figures in the paper, to make sure that the ideas are really being expressed clearly. It was a pleasure having her as part of the team and I’m personally really excited that we got the result we did,” said Lalor.
@nditaodept
@nditaodept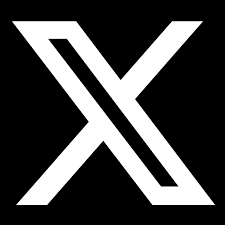
YouTube Channel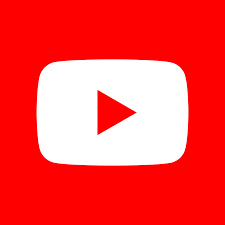
LinkedIn ITAO Group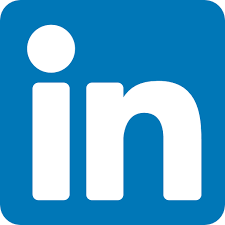
Check out these featured stories:
Topics: